Improving Metropolitan-Scale Transportation Systems
|
NSF CNS-1446640 |
Project Introduction
Under the Traditionally, the design of urban transit services has been based on limited sampling data collected through surveys and censuses, which are often dated and incomplete. The theory and practice of transit services have also typically focused on isolated individual transportation modes. These two limitations result in unsatisfactory passenger experiences, such as unnecessary detours and prolonged travel delays. Fortunately, a new opportunity to address these limitations has arisen in recent years: the latest expansion of urban information infrastructure enables us to model behaviors of urban transportation systems with massive multi-modal online feeds and to apply effective local and global cyber-control. Our work will transform the efficiency of existing transportation systems because (i) in addition to macro-level historical statistics, the availability of massive micro-level trip information will make it possible to apply fine-grained real-time control to handle rapid changes in dynamic urban environments and (ii) aggregated information from multi-modal transit allows more effective inter-transit coordination.
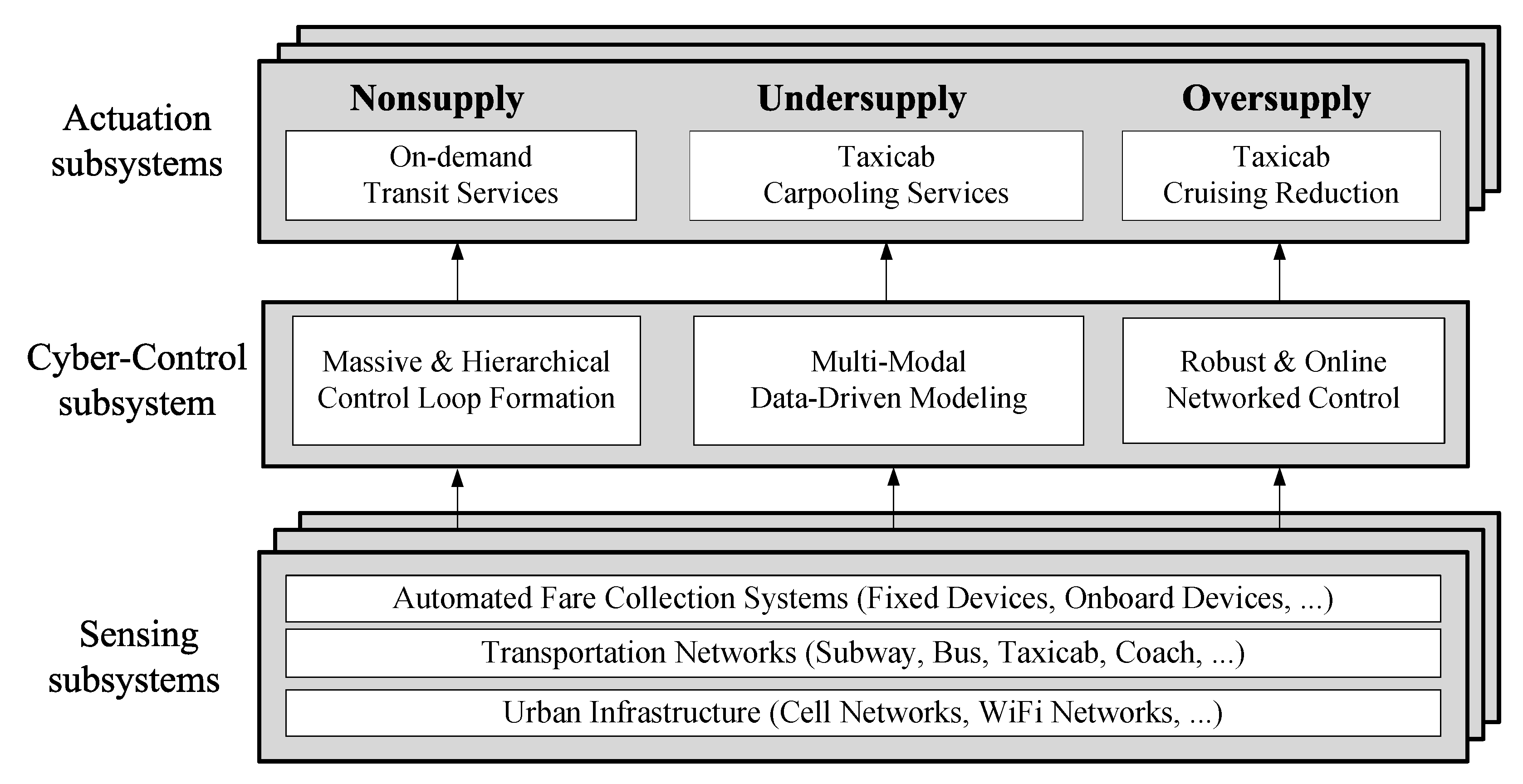
Challenges
From a cyber-control perspective, our research is driven by large-scale online multi-modal transit data to achieve equilibrium in demand and supply relationship in three dynamic urban scenarios:
Nonsupply: Urban public transit systems (e.g., bus and subways) contribute significantly to the reduction of congestion, but they typically stop at long intervals. Consequently, many urban locations are beyond walking distance of the existing public transit supply, an issue known as the ”last mile problem”. Accordingly we propose a minibus service to deliver passengers from public transit stations to their final destinations using data-driven cyber-control, addressing challenges in three key aspects: coverage, frequency, and capacity.
Undersupply: During rush hours, urban transit services become insufficient. To address the undersupply issue, we propose to investigate the benefits, opportunities, economic incentives, and practical mechanisms of a taxi pooling cyber-control system. We will address key challenges related to spatiotemporal dynamics in both supply and demand and coexistence of taxi pooling with regular taxi service.
Oversupply: After rush hours, the transit supply become more than sufficient. As a result, taxi drivers are forced to spend excessive time and gas to find their next passenger, an issue we refer as the “high cruising mile problem”. We propose to investigate cyber-control to navigate vacant taxis, based on feeds automatically collected from other roving vacant taxis and urban infrastructure (e.g., cellular stations), addressing challenges on data-driven demand modeling, hierarchical control, and coordination.
Based on three scenarios, we expect that generic principles and design methodologies will emerge and guide us to build an integrated Transportation Cyber-Control System (TCCS), which balances demand-and-supply relationships across multiple transit modes and regions.
Demos
|
Carpooling Taxicab ServicesThis 3 min video presents the basic idea of our real-time carpooling system. Carpooling taxicab services hold the promise of providing additional transportation supply, especially in the extreme weather or the rush hour when regular taxicab services are insufficient. Although many recommendation systems about regular taxicab services have been proposed recently, little research, if any, has been done to assist passengers to find a successful taxicab ride with carpooling. In this paper, we present the first systematic work to design a unified recommendation system for both the regular and carpooling services, called CallCab, based on a data driven approach. In response to a passenger's real-time request, CallCab aims to recommend either (i) a vacant taxicab for a regular service with no detour, or (ii) an occupied taxicab heading to the similar direction for a carpooling service with the minimum detour, yet without assuming any knowledge of destinations of passengers already in taxicabs. |
|
Carpooling in New York CityThis 1 min video visualizes the New York City taxi system with our carpooling services about 13,605 taxicabs on Jan 7 2013. It renders the reduced cumulative mileage and traffic because of taxi carpooling in NYC. The top figure gives real-time mileages for both the ground truth and carpooling. The two maps show the ground truth of pickup and dropoff patterns as well as carpooled pickup and dropoff patterns, indicating reduced traffic. |